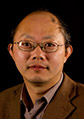
Yonghuai Liu
Senior Lecturer, Aberystwyth University, UK
Title: 3D shape matching for object modelling
Biography
Biography: Yonghuai Liu
Abstract
3D data can be easily captured nowadays using the latest laser scanners such as Microsoft Kinect. Since the scanners have limited field of view and one part of an object may occlude another, the captured data can only cover part of the object of interest and is usually described in the local scanner centred coordinate system. This means that multiple datasets have to be captured from different viewpoints. In order to fuse information in these datasets, they have to be registered into the same coordinate system for such applications as object modelling and animation. The purpose of scan registration is to estimate an underlying transformation so that one scan can be brought into the best possible alignment with another. To this end, various techniques have been proposed, in which the feature extraction and matching (FEM) is promising due to its wide applicability to different datasets subject to different sizes of overlap, geometry, transformation, imaging noise, and clutters. In this case, the established point matches usually include a large proportion of false ones.
3D data can be easily captured nowadays using the latest laser scanners such as Microsoft Kinect. Since the scanners have limited field of view and one part of an object may occlude another, the captured data can only cover part of the object of interest and is usually described in the local scanner centred coordinate system. This means that multiple datasets have to be captured from different viewpoints. In order to fuse information in these datasets, they have to be registered into the same coordinate system for such applications as object modelling and animation. The purpose of scan registration is to estimate an underlying transformation so that one scan can be brought into the best possible alignment with another. To this end, various techniques have been proposed, in which the feature extraction and matching (FEM) is promising due to its wide applicability to different datasets subject to different sizes of overlap, geometry, transformation, imaging noise, and clutters. In this case, the established point matches usually include a large proportion of false ones.
This talk will focus on how to estimate the reliability of such point matches from which the best possible underlying transformation will be estimated. To this end, I will first show some example 3D data captured by different scanners, from which some issues can be identified that the registration of multiple scans is challenging. Then I will review the main techniques in the literature. Inspired by the AdaBoost learning techniques, various novel algorithms will be proposed, discussed and reviewed. These techniques are mainly based on the real and gentle AdaBoost respectively and include several steps: weight initialization, underlying transformation estimation in the weighted least squares sense, estimation of the average and variance of the errors of all the point matches, error normalization, and weight update and learning. Such steps are iterated until either the average error is small enough or the maximum number of iterations has been reached. Finally, the underlying transformation is re-estimated in the weighted least squares sense using the weights estimated.
I will thirdly validate the proposed algorithms using various datasets captured using Minolta Vivid 700, Technical Arts 100X, and Microsoft Kinect and show the experimental results. To show the robustness of the proposed techniques different FEM methods will also be considered for the establishment of the potential point matches: signature of histograms of orientations (SHOT) and unique shape context (USC), for example. Finally, I will conclude the talk and indicate some future work.I will thirdly validate the proposed algorithms using various datasets captured using Minolta Vivid 700, Technical Arts 100X, and Microsoft Kinect and show the experimental results. To show the robustness of the proposed techniques different FEM methods will also be considered for the establishment of the potential point matches: signature of histograms of orientations (SHOT) and unique shape context (USC), for example. Finally, I will conclude the talk and indicate some future work.